Nowadays, power forecasting has already become a vital component of the energy market. Being able to know if generation will be enough to match demand is no longer a convenience, but a necessity. However, the difficulty associated with executing a precise forecast varies in function of which technology is used to produce that power, and it is widely accepted that power generation from solar energy occupies a top spot on the list.
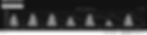
Given the large number of countries drawing a considerable amount of their energy from solar power, irradiance forecasting has become a top-priority technology, with the potential of affecting the efficiency of the entire energy market. This is explained in the context where accurate predictions are a key point for efficient management, operation strategies and solar energy trading.
Although they share a common goal, there are several methods for achieving proper forecasting of solar irradiance. In this article all such methods are analyzed in order to fully understand their precision, sensitivity and interaction capabilities.
Sky imagers
Sky imagers are obtained though the utilization of digital cameras providing high-quality images from horizon to horizon. These pictures are then used for detecting clouds, estimating their distance from the ground and calculating their motion (speed and direction). Since not all clouds distort irradiance to the same degree, sky imagers are also used to determine the influence these clouds will have on the final solar power potential. The application of this method is limited to a short horizon forecast due to its fall in accuracy beyond 30-minute time frame. This is why this method is considered expensive to use relative to the other short-term prediction methods.
Satellite Imagery
Satellite Imagery provides a longer look-ahead time frame. This method is engaged by a first stage where a physically-driven model is used to predict clear sky conditions at a given site. After that, this model is successively modulated by the estimated irradiance calculated from satellite images. When counting on a sequence of images taken by the satellite, they can also be used to create a combined model to predict future cloud formation spots.
Satellite Imagery has proved its effectiveness in forecasting irradiance from one minute and up to five hours ahead. However, its performance is poor when facing conditions where the clouds are either forming or dissipating rapidly. This includes marine cloud regimes, among others.
Numerical Weather Prediction with Model Output Statistics
Numerical Weather Predictions (or NWPs) have been the flagship of forecasting applications for many years. They hold the highest accuracy for periods starting from six hours and up to two weeks. They also had been reinforced by recently deployed rapid-update systems that shorten their time scales, improving the overall results. NWP methods also play a vital role in Wind Power forecasting.
Variables that have been historically included in these models’ calculations include temperature, humidity, precipitation probability and wind conditions. NWP methods are usually complemented by Statistic Learning methods, which help in correcting and polishing errors, araising the precision of NWP models by about 10-15%.
Statistical Learning methods
Statistical Learning models are widely applied for solar power forecasting. They are engaged by a training phase where they learn from historic data, building the patterns that they’ll consider valid and worth applying in future calculations. Statistical learning methods include a diverse spectrum of approaches, running from classical regression methods to the more complex heights of artificial neural networks.
An advantage of these methods against others is that they can be used to derive irradiance forecasts without need for any physical modeling. Also as said before, they play a key role in strengthening the performance of NWP methods, forming a communion that usually receives the name of statistical post processing or model output statistics.
Evaluation of Results
Once the models have been run and the results are obtained, their quality and reliability are evaluated by comparing them to reference data. Irradiance measurements obtained at ground level are commonly used for that purpose. Forecast evaluation provides a report of the overall accuracy of the model or combination of methods used. This information is meant to aid the user in decision making rewarding whether the model being used is the optimal for the needed conditions (desired response time, forecast horizon…).
Executing a detailed evaluation is crucial for model testing and further model development. In this way, future predictions will benefit from what the model was able to learn and overall accuracy will increase.
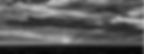
Future of Solar Forecasting
Solar forecasting can still be considered a new technology. Thus, there is a a wide margin for improvement in its accuracy. Probabilistic forecasting techniques that could contribute to reducing uncertainty are still under development. Most of the routes that are being explored, however, involve the interaction of the previous methods in order to generate synergies between the unique advantages of each one. In this way, the performance of Solar Forecasting Methods will keep improving over time, enabling them to make an invaluable contribution to the energy market.